Bias and Fairness in AI: The Challenge of Removing Unwanted Biases
Discover the imperative role of technology in combatting bias within AI systems. From mitigating unfair discrimination to ensuring ethical outcomes, explore how Balnc's innovation reshapes the landscape of unbiased artificial intelligence.
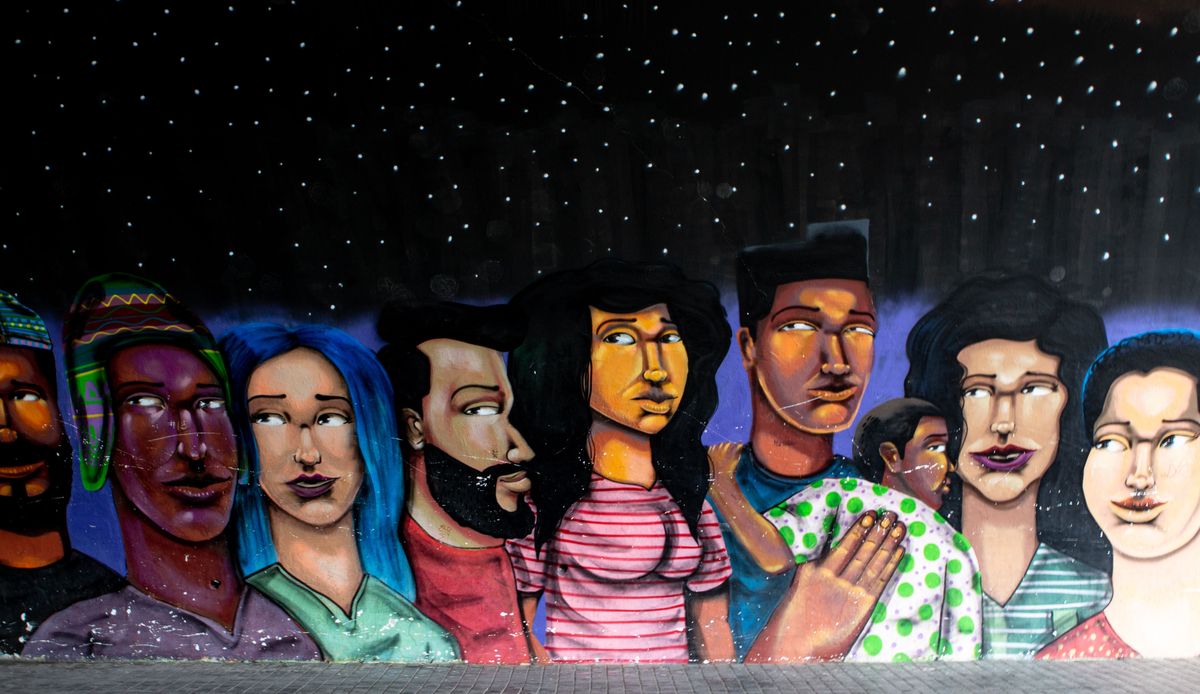
Artificial Intelligence (AI) is becoming an integral part of our daily lives, from influencing the content we see on social media to determining loan eligibility or even guiding autonomous vehicles. However, the rise of AI has brought to light a significant challenge that we must address: bias in machine learning models. In this in-depth article, we will explore the issue of bias in AI, its implications, and the ongoing efforts to remove these unwanted biases. We will also delve into case studies, examples, and fact-checking to provide a comprehensive understanding of this complex issue.
The Bias Dilemma
Machine learning models, including large language models, computer vision systems, and recommendation algorithms, learn from data. This data, often collected from real-world sources, can contain biases that are present in society. As a result, machine learning models may inadvertently learn and perpetuate these biases, leading to biased decisions and outcomes. Bias in AI can manifest in various forms, including gender, race, age, socioeconomic status, and more.
Bias in AI can have serious consequences. It can lead to unfair or discriminatory outcomes, reinforcing stereotypes and exacerbating societal inequalities. For instance, an AI-based hiring system that favors male candidates over female candidates can perpetuate gender bias in the workplace. Similarly, a criminal justice system that uses biased algorithms for risk assessment can disproportionately impact minority communities.
The issue of bias in AI is complex and multifaceted, making it a critical challenge that must be addressed in the development and deployment of AI systems.
Identifying Bias in AI
Before we dive into the efforts to remove bias, let's understand how we can identify it in AI systems. Bias can manifest in various ways:
- Input Bias: Bias in the data used to train the model. For example, if a facial recognition system is trained primarily on data containing images of lighter-skinned individuals, it may perform poorly on darker-skinned individuals.
- Algorithmic Bias: Bias introduced by the design and algorithm choices of the model. This could be due to skewed sampling, features used, or the optimization process.
- User-Induced Bias: Bias introduced by users' interactions with the system. For instance, a recommendation algorithm that suggests content similar to what a user has previously engaged with may inadvertently reinforce biases.
Case Studies: Real-World Consequences
To understand the gravity of bias in AI, let's explore a few case studies that highlight the real-world consequences.
Case Study 1: Biased Criminal Risk Assessment
In the United States, some states have adopted risk assessment tools to aid judges in determining bail and sentencing decisions. These tools are designed to be objective, but they have been criticized for perpetuating racial bias. A ProPublica investigation found that a widely used risk assessment tool incorrectly labeled black defendants as high risk at nearly twice the rate as white defendants, while it misclassified white defendants as low risk more often than black defendants.
This case study demonstrates how biased algorithms can have a profound impact on individuals' lives, particularly in the criminal justice system.
Case Study 2: Gender Bias in Language Models
Language models like GPT-3 have been trained on vast amounts of text from the internet. While this enables them to generate human-like text, it also exposes them to the biases present on the web. OpenAI, the organization behind GPT-3, has acknowledged that their model sometimes produces outputs that exhibit gender bias. For instance, when prompted with certain phrases, the model may generate sexist or discriminatory content.
This case study underscores the challenges of addressing bias in large language models and the need for ongoing refinement.
Addressing Bias in AI
The fight against bias in AI is a multifaceted effort that involves researchers, policymakers, and technology companies. Here are several strategies and initiatives aimed at addressing and removing bias:
1. Data Collection and Curation:
- Diverse Data: Collect diverse and representative data to ensure that the training data does not reinforce existing biases. This involves actively seeking data from underrepresented groups.
- Data Auditing: Regularly audit and review the training data to identify and rectify bias. This process involves working with human annotators to classify and correct data points.
2. Algorithmic Fairness:
- Fairness Metrics: Develop and apply fairness metrics to evaluate the performance of AI systems. These metrics can help identify disparities in outcomes based on various demographic factors.
- Bias Mitigation Techniques: Implement bias mitigation techniques in algorithm design and optimization. These techniques aim to reduce disparate impacts on different groups.
3. Transparency and Explainability:
- Model Explainability: Develop methods to make AI models more transparent and interpretable, allowing users to understand how decisions are made.
- External Auditing: Engage external organizations to conduct audits of AI systems to assess and report on their fairness and bias mitigation efforts.
4. User Feedback and Iteration:
- Feedback Loops: Create mechanisms for users to provide feedback on biased content or outcomes. These feedback loops can inform model improvements.
- Continuous Iteration: Regularly update and refine AI models based on feedback and new data to reduce bias.
Fact-Checking and Accountability
Ensuring fairness and mitigating bias in AI requires rigorous fact-checking and accountability measures. Technology companies, regulatory bodies, and the wider AI community need to collaborate to:
- Fact-Check Models: Conduct regular audits of AI models to assess their performance and identify bias. Independent organizations can play a crucial role in this process.
- Transparency Reports: Release transparency reports that detail the steps taken to address bias, as well as the progress made in removing unwanted biases from AI systems.
- Accountability for Harm: Hold technology companies accountable for the harm caused by biased AI systems. This can involve regulatory measures and legal consequences for negligence in addressing bias.
Conclusion
Bias in AI is a pervasive issue that affects various aspects of our lives. Its consequences are far-reaching, from discriminatory hiring practices to reinforcing societal stereotypes. Efforts to address and remove bias in AI are ongoing, with researchers and organizations actively working to improve the fairness of AI systems.
As we move forward, it is crucial to combine technological innovation with ethical considerations. Removing bias in AI is not a one-time endeavor but an ongoing commitment to create AI systems that are fair, transparent, and equitable.
In conclusion, the journey to remove bias in AI is a complex and dynamic one, but it is essential for building AI systems that are both intelligent and just.